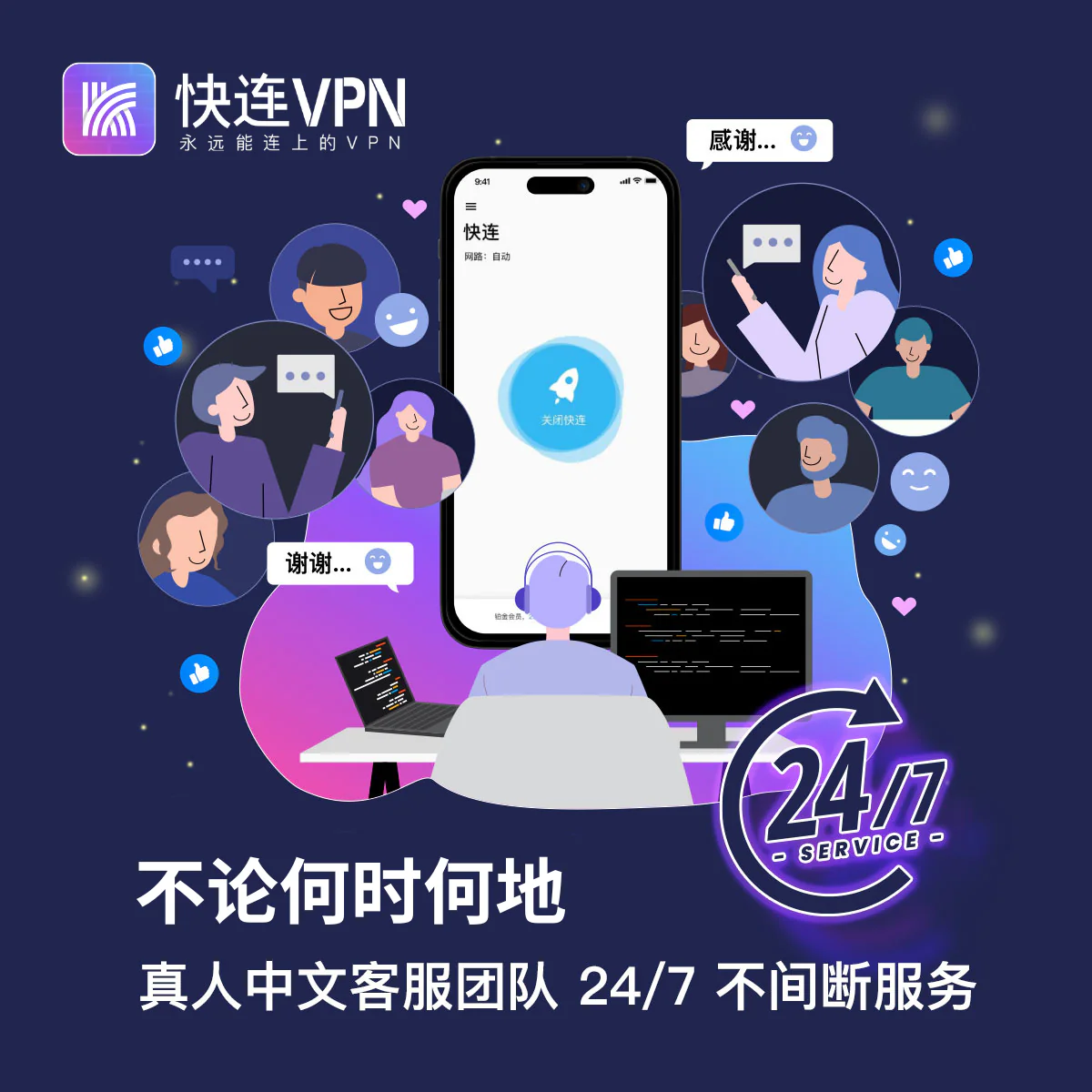
有效的工作流程編排是在現代軟件開發環境中圍繞複雜的面向流程的活動創建自動化的關鍵。考慮到數據工程和數據科學,astro 和 apache airflow 作爲管理這些數據工作流的重要工具名列前茅。
本文比較了 Astro 和 Apache Airflow,解釋了它們的架構、功能、可擴展性、可用性、社區支持和集成功能。這應該有助於軟件開發人員和數據工程師根據他們的特定需求和項目要求選擇正確的工具。
Astro 概述
Astro 是一個完全 Kubernetes 原生的平臺,旨在輕鬆編排以下工作流程雲原生系統。它使用 Kubernetes 本身來處理容器編排,這增加了開箱即用的容錯能力和彈性。因此,Astro 在需要微服務和容器化對架構至關重要的場景中有效工作。
特性和功能
Astro 提供了一種定義工作流程的聲明式方法,可以在 Python 中定義或 YAML。同時,它簡化了對 Kubernetes 的接口負擔。此外,Astro 還管理動態擴展所需的資源。 Astro 原生地與現代數據結構配合使用 - 開箱即用 - Kubernetes Pod,使數據庫、雲服務和處理數據的框架之間的通信更加輕鬆。
示例代碼片段
dag_id: first_dag # This is the unique identifier for the DAG.schedule: "0 0 * * *" # This specifies the schedule for the DAG using a cron expression (runs daily at midnight).tasks: # This is the list of tasks in the DAG. - task_id: my_task # This is the unique identifier for the task. operator: bash_operator # This specifies the type of operator to use (in this case, a BashOperator). bash_command: "echo Welcome to the World of Astro!" # This is the command that will be run by the BashOperator.登錄後複製
Apache Airflow 概述
Apache Airflow 是一個開源平臺,最初由 Airbnb 開發,由於其可擴展性、可擴展性和豐富的功能而被廣泛採用。與僅在 Kubernetes 上運行的 Astro 不同,Airflow 的架構通過 DAG 定義工作流程。它將任務的定義與執行分開,因此允許在節點集羣中以分佈式方式執行任務。
特性和功能
Airflow 基於 Web 的 UI 提供任務依賴項,執行狀態和日誌,使其在調試和監控時更加高效。它也適用於大多數工作流程要求;它有大量可用於任務的運算符,範圍從 Python 腳本到 SQL 過程或 Bash 命令等。然後,插件設計通過向廣泛的雲服務、API 和數據源開放,使 Airflow 變得更加強大。
示例代碼片段
from airflow import DAG # Importing DAG class from Airflowfrom airflow.operators.bash_operator import BashOperator # Importing BashOperator classfrom datetime import datetime, timedelta # Importing datetime and timedelta classesdefault_args = { 'owner': 'airflow', # Owner of the DAG 'depends_on_past': False, # DAG run does not depend on the previous run 'start_date': datetime(2023, 1, 1), # Start date of the DAG 'retries': 1, # Number of retries in case of failure 'retry_delay': timedelta(minutes=5), # Delay between retries}dag = DAG('first_dag', default_args=default_args, schedule_interval='0 0 * * *') # Defining the DAGtask_1 = BashOperator( task_id='task_1', # Unique identifier for the task bash_command='echo "Welcome to the World of Airflow!"', # Bash command to be executed dag=dag, # DAG to which this task belongs)登錄後複製
比較
可擴展性和性能
Astro 和 Apache Airflow 在可擴展性方面都很強大,但方式不同但又相關。另一方面,Astro 非常好地利用 Kubernetes 架構,通過動態管理容器來實現水平擴展,非常適合彈性擴展。 Airflow 藉助分佈式任務執行模型實現了擴展,該模型可以在許多工作節點上運行,並提供管理大規模工作流程的靈活性。
易用性和學習曲線
Astro 與 Kubernetes 的集成可能會讓那些熟悉容器編排的人輕鬆部署,但這可能會給那些剛接觸容器概念的人帶來更陡峭的學習曲線庫伯內斯。相反,Airflow 配備了非常友好的 Web 界面和豐富的文檔,使入門變得簡單,並且任務定義和執行之間清晰分離 - 更加用戶友好,使工作流程管理和故障排除更加簡單。
社區和支持
廣泛的支持、持續的開發以及龐大的插件和集成生態系統使該項目能夠通過支持 Apache Airflow 的龐大、充滿活力的開源社區不斷改進和創新。作爲一個比其他解決方案更新且不太成熟的解決方案,Astro 背後的社區較小,但爲企業部署提供專業的支持選項。它在社區驅動的創新和企業級可靠性之間實現了良好的平衡。
集成能力
Astro 和 Apache Airflow 都與大量數據源、數據庫和雲平臺相結合。 Astro 原生與 Kubernetes 集成,允許在也支持 Kubernetes 的雲系統上順利部署,從而提高其與其他雲原生服務和其他工具的互操作性。 Airflow 集成的力量通過其插件生態系統擴展到 Airflow 用戶,輕鬆將管道連接到任何數據源、API 和雲服務。
結論
選擇 Astro 或Apache Airflow 需要特定的項目需求、基礎設施喜好,最後還需要團隊技能。得益於 Astro 以 Kubernetes 爲中心的方法,該工具仍然是容器化和微服務架構的絕佳解決方案,旨在在雲原生環境中提供可擴展且高效的工作負載。另一方面,Apache Airflow 成熟的生態系統、廣泛的社區支持和非常靈活的架構使其成爲真正需要跨不同數據管道進行強大的工作流程編排的團隊的必備解決方案。
瞭解其威力和微妙之處每個工具的功能允許軟件開發人員和數據工程師按照組織目標和技術要求的方向做出決策。隨着數據工程和軟件開發空間的不斷擴大,Astro 和 Apache Airflow 再次不斷發展,提供最能滿足現代工作流程需求的解決方案。
以上就是Apache Astro 和 Airflow 的比較的詳細內容,更多請關注本站其它相關文章!